Vox Harmoniser
1. A description of the creative motivation and intended use (if applicable) of your project. Why did you make it? Who do you envision using it, and where/when/why?
My project is inspired by our ability to experiment with, mould and shape the human voice using technology. As a vocalist, I have always been passionate about exploring the possibilities of the voice, and the role that technology can play in enhancing and expanding our capacity to create sound is an area I have delved into in a musical context for many years. Through studying Machine Learning, I wanted to create a tool that would harmonise with me as I sung, and feel quite intuitive yet also somewhat autonomous. As a film composer, I intend to use my Vox Harmoniser to create scores, and the ability to program harmonic flexibility means that it could be used in a multitude of musical styles and frameworks. It could also be quite interesting in a live context, somewhat like a vocoder but without the need to play chords in real time, which would provide some freedom and could potentially insert surprises.
2. How does your project relate to existing work? (Cite existing academic and artistic work using academic references, and provide URLs to existing work on the web.)
Matthew Herbert’s work is a great inspiration. He often explores layering and experimenting with the human voice, not necessarily using technology but always in inventive and sometimes strange ways (https://wellcomecollection.org/chorus).
I recently saw Holly Herndon and Mat Dryhurst talk at CTM in Berlin, and their project Spawn is quite relevant to the conceptual framework of the Vocal Harmonium. Spawn is a neural net that Herndon and Dryhurst are training to recognise their voices as parents, with the goal of Spawn creating sound and music after enough training examples and time. (https://www.berlin-ism.com/en/news/holly-herndon-mathew-dryhurst).
Probably the most well known example of Machine Learning for gestural music is Imogen Heap’s Mi.Mu gloves, which allow her to live sample and utilises Machine Learning to break a stage space up into quadrants that control different musical aspects for her to engage with gesturally. (https://www.youtube.com/watch?v=7oeEQhOmGpg).
Scott Tooby created a really amazing harmoniser tool, which I found to be an excellent example of what is possible using Wekinator and SuperCollider. (https://github.com/stooby/SCML) (https://vimeo.com/185095490).
There is much academic research on extracting the human voice in audio files and ways to recognise speech, which have had obvious economic and social impacts already, considering the prevalence of Siri, Cortana and other virtual assistants. However, there is not a huge amount of artistic experimentation when it comes to combining Machine Learning and the voice.
I found an interesting joint study between the Centre for Digital Music at Queen Mary University of London and the Music and Audio Research Laboratory, New York University which utilised Machine Learning to analyse and characterise singing styles from around the world. (http://www.eecs.qmul.ac.uk/~simond/pub/2017/PanteliBittnerBelloDixon-ICASSP.pdf).
+ M Panteli, R Bittner, JP Bello, and S Dixon. Towards the characterization of singing styles in world music. In ICASSP, IEEE International Conference on Acoustics, Speech and Signal Processing - Proceedings, pages 636 - 640, Jun 2017.
I also found a study of personalised voice synthesis using unsupervised Machine Learning in the Computer Music Journal by the Faculty of Engineering and Information Sciences University of Wollongong in Dubai and the Communication and New Media Department National University of Singapore. The study created a method that allowed users to synthesise their voice based on timbral quality and change over time, with the intention of hiding parameters that would be recognisably synthesis-related, focusing on establishing a perceptual experience. (https://www.mitpressjournals.org/doi/abs/10.1162/comj_a_00450).
+ Fasciani S, Wyse L. Vocal Control of Sound Synthesis Personalised by Unsupervised Machine Listening and Learning. Computer Music Journal, Volume 42, Issue 1, pages 37 - 59, 2018.
3. A description of how you implemented the project. What machine learning and/or data analysis techniques have you used, and why? What software tools or libraries did you use? What datasets did you use?
I paired Wekinator with Max/MSP to implement the Vox Harmoniser which has been a relatively seamless combination. With Scott Tooby’s example in mind, I created a simple polyphonic synth in Max, and built that into a patch that sends and receives OSC from Wekinator. I created 12 channels, 1 for each note in a chromatic C scale, and mapped the midi notes for each. Using the bark extractor example from the Wekinator site, and Tristan Jehan’s analyzer~ and OpenSoundControl objects (developed at CNMAT), I was able to analyse audio through either my computer’s built in mic or an external microphone and an audio interface. I then started designing chords that would be triggered by specific notes when sung, and realised early on that I would need a lot of training sets to firstly, experiment and iron out any weird glitches, but also to extend the capability of the tool so that it could be used in many keys and modes.
I set out to train Wekinator using classification for a couple of reasons: firstly, I wanted definition between my training examples; secondly, I decided to use midi to create a sonically pleasing tool that would fit into equal temperament Western music, and as such, classification seemed like the best option, or else I would have had to create each note using frequency, which may have been quite unwieldy when running the program, and couldn’t easily be paired with other instrumentation.
I wanted to experiment with the classification algorithms quite a lot to see if any were more suited to the project. I started with k-Nearest Neighbour, then experimented with AdaBoost, which both seemed to react similarly. They were relatively responsive and quite accurate. I then worked with the Decision Tree algorithm, which jumped around a lot more and made for a more unstable listening experience. Next, I tried SVMs with a polynomial kernel, which was quite unruly, and chose to play only a couple of notes from each chord at random — definitely not a stable choice. I then explored SVMs with a linear kernel which seemed even more unruly, and Naive Bayes didn’t even recognise silence, despite me adding many extra training examples. I went back to AdaBoost (using a base classifier of Decision Tree), but later decided the speedy training and responsiveness of k-Nearest Neighbour was the best fit.
Considering how unstable classifying the human voice can be, I was happily surprised by the output. If it becomes a tool that many people interact with, it would require training sets that involve voices of as many timbres and registers as possible.
4. A reflection on how successful your project was in meeting your creative aims. What works well? What challenges did you face? What might you change if you had more time?
The project mainly involved creating multiple Max patches to send OSC to Wekinator and then receive OSC from Wekinator to process the synthesis. The signal processing element of the project worked very well, more seamlessly than expected, which was great. The communication between Max/MSP and Wekinator is really reliable and that has made the unpredictability of training on the human voice a little more stable. Creating sets of chords that sounded harmonically pleasing was one of the more difficult and time-consuming aspects of the project, as it required composing chords, training and running to discover whether or not it was a training set worth keeping. Given more time, I would make larger training sets and source training sets from people with different vocal ranges and timbres to maximise the project’s scalability. I would love to have different modes and keys to choose from so that you could improvise within specific musical contexts in a cohesive way. It would also be fun to expand the signal processing effects, creating different filters, reverbs and delays depending on the frequency, amplitude or timbre of the input.
5. A clear statement of which third-party resources (e.g., code, data) you have used, and a statement of which aspects of the project are your own work.
I used a Max/MSP patch from the Wekinator website in the Audio Downloads section called Bark Extractor, which extracts 25 bark coefficient inputs and communicates these to Wekinator over OSC (http://www.wekinator.org/examples/#Audio). I used Tristan Jehan’s analyzer~ and OpenSoundControl objects, developed at CNMAT (http://cnmat.berkeley.edu/downloads), which were required to utilise the Bark Extractor patch. I wrote the main Vox Harmoniser patch, as well as the octave subpatch, containing each potential midi note required over 4 octaves. I created a polyphonic synth subpatch called Synthy, and the synth voices inside another subpatch called Synthy_Voice using this Cycling 74 tutorial (https://cycling74.com/tutorials/polyphonic-synthesizer-video-tutorial). I then created the Wekinator project to train and run the program and used Metric Halo, my audio interface, to capture and minimally mix the audio.
6. Instructions for compiling and running your project.
Open the Wekinator project called "VoxHarmoniserMajor TO USE". Also open the Max/MSP VoxHarmoniser patch, and the Bark Extractor patch (both will have the DAC on when you’re running it, that is fine). You will need headphones and either an audio interface to route the audio, or you can user your internal computer microphone and speakers. Without headphones on, the harmoniser will create a feedback loop. Turn the DAC on in the Vox Harmoniser patch, run the Wekinator project and make some noises. Voila!
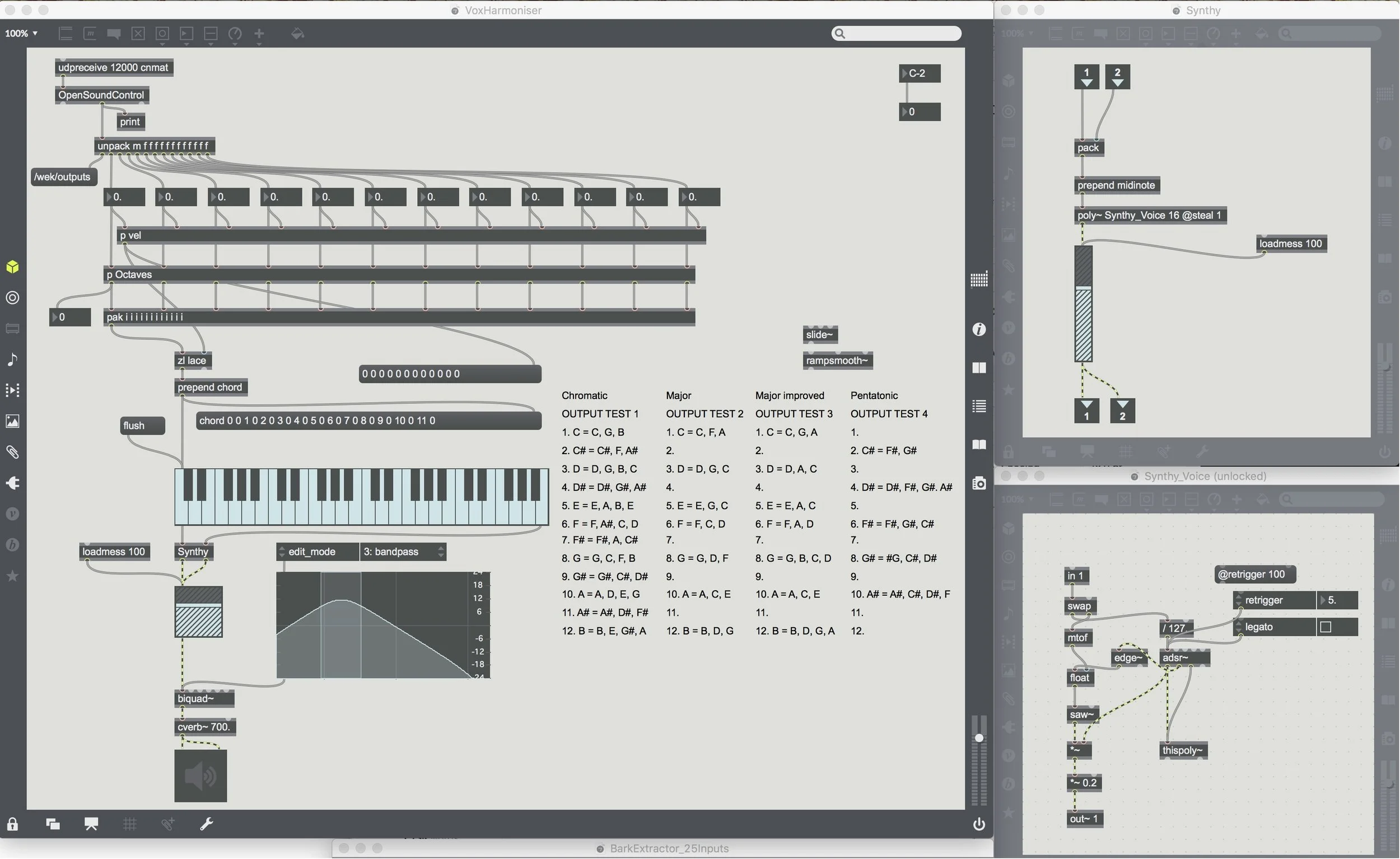
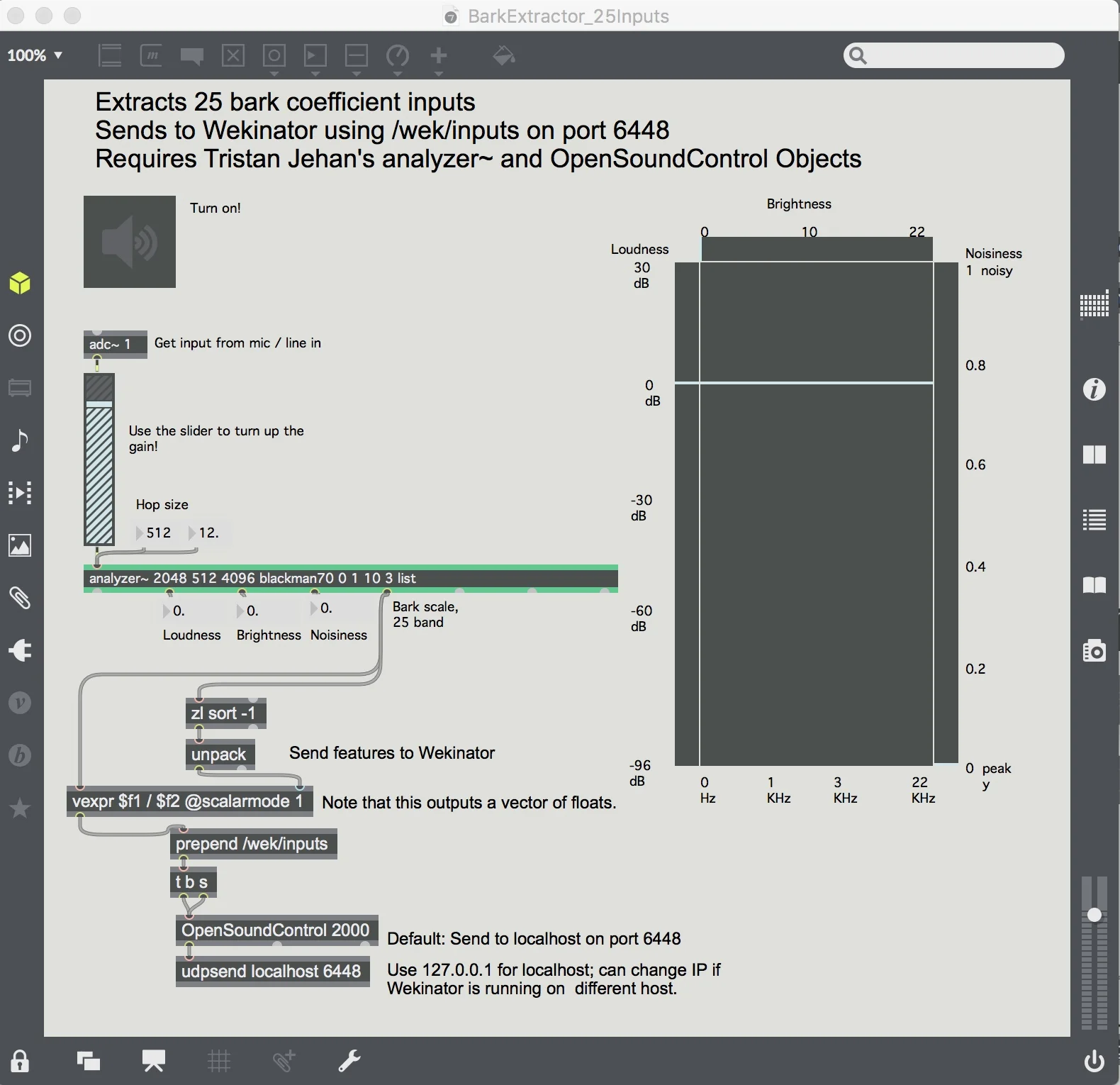
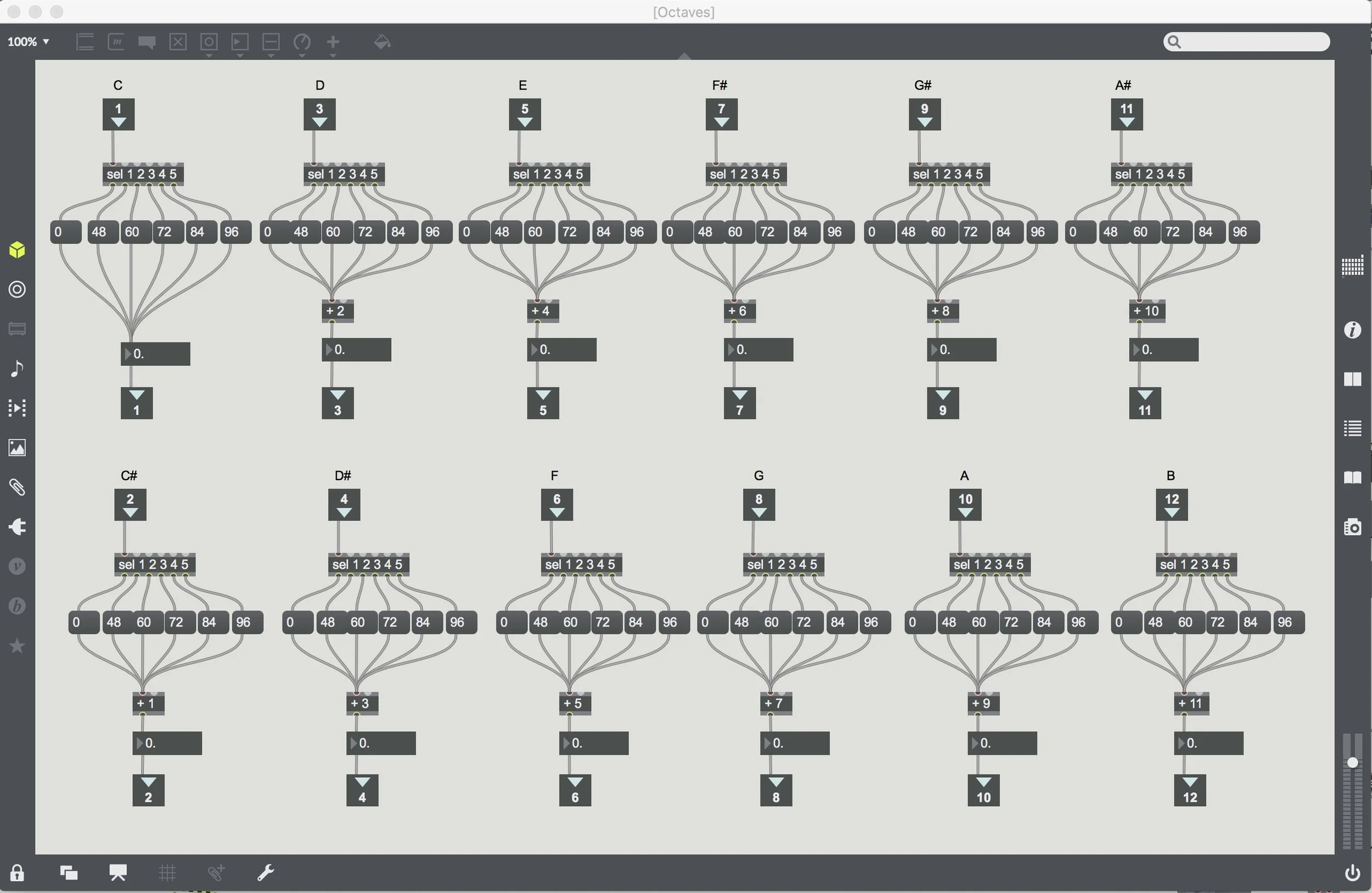
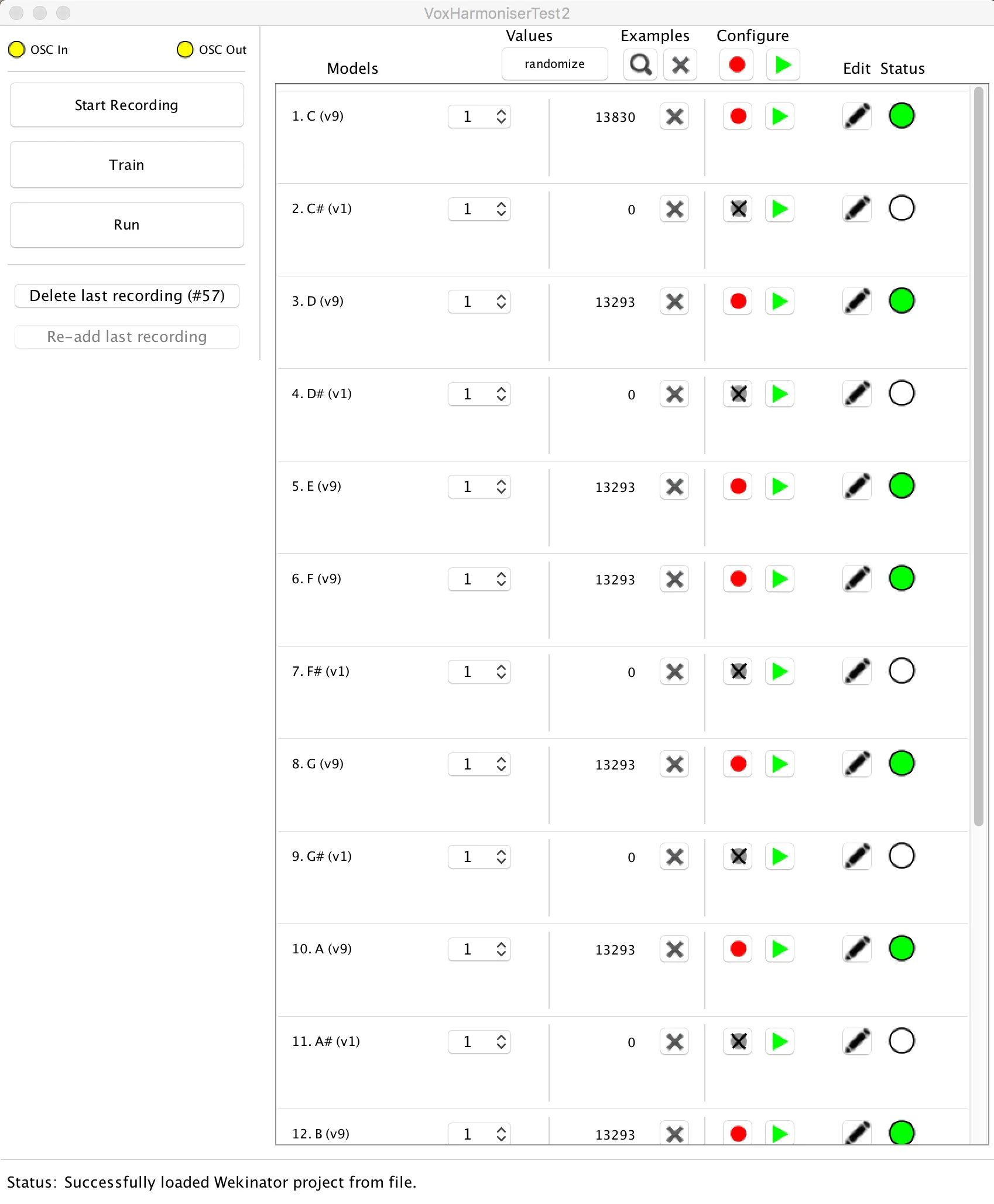